PM2.5 CONCENTRATION PREDICTION AND UNCERTAINTY ANALYSIS BASED ON A COMPOSITE MODEL
-
摘要: 运用GIS软件及克里金(Kriging)插值等方法分析合肥城市圈PM2.5浓度的时空分布,根据合肥市环境监测历史数据、地面气象站点数据及历史气象数据,采用多元回归分析、相关分析等方法,研究合肥市PM2.5浓度的影响因素。结果表明:1)PM2.5 浓度整体变化情况为冬季 > 秋季 > 春季 > 夏季,大部分城市PM2.5浓度峰值出现在1月,之后浓度开始逐渐下降,7月达到最低值,此后浓度逐渐升高。2)PM2.5浓度与CO呈高度正相关,相关系数高达0.875;与PM10、SO2、NO2的相关性也较高;与O3呈负相关。PM2.5浓度与气压、风速、降雨量以及能见度呈负相关,与温度、相对湿度呈强正相关。基于2018—2019年合肥市地面站点PM2.5浓度监测数据,构建预测PM2.5浓度的组合模型:对比三次指数平滑模型,确定模拟退火+遗传+三次指数平滑为优组合模型,拟合度达到95%。通过Kappa及MAPE指数对组合模型不确定性进行分析评价,两者分别为0.654和0.072,说明该模型具有高度稳定性。恰当的预测因子组合和模型不确定性研究有助于模型预测精度的提升和改善,从而为大气环境质量监测和评价提供参考。Abstract: In this paper, GIS software and Kriging interpolation method were used to analyze the spatial and temporal distribution of PM2.5 concentration in Hefei city circle. According to historical environment monitoring data, ground meteorological stations and historical meteorological data of Hefei, multiple regression analysis, correlation analysis and other methods were adopted to study the influencing factors of PM2.5 concentration in Hefei. The results showed that: 1) the overall change of PM2.5 concentration in the above cities was in the order of winter > autumn > spring > summer, and PM2.5 concentration in most cities peaked in January, then gradually declined, reached the lowest value in July, and then gradually increased; 2) PM2.5 concentration showed a highly positive correlation with CO, with the correlation coefficient as high as 0.875. The correlation with PM10, SO2 and NO2 was also high. There was a negative correlation with O3. PM2.5 concentration was negatively correlated with air pressure, wind speed, rainfall and visibility, and strongly positively correlated with temperature and relative humidity. Based on PM2.5 concentration monitoring data of Hefei from 2018 to 2019, a composite model was built to predict PM2.5 concentration, and three times exponential smoothing model was compared to determine that simulated annealing+genetic+three times exponential smoothing was the optimal composite model, with the fitting degree reaching 95%. Kappa and MAPE indexes were used to analyze and evaluate the uncertainty of the composite model. Kappa and MAPE indexes were 0.654 and 0.072 respectively, indicating that the model was highly stable. The proper combination of prediction factors and the study of model uncertainty were helpful to improve the model prediction accuracy, so as to provide theoretical basis and method for the monitoring and evaluation of atmospheric environment quality.
-
郭家瑜,张英杰,郑海涛,等.北京2015年大气细颗粒物的空间分布特征及变化规律[J].环境科学学报,2017,37(7):2409-2419. 姜磊,周海峰,赖志柱,等.中国城市PM2.5时空动态变化特征分析:2015-2017年[J].环境科学学报,2018,38(10):3816-3825. 李大年,余家燕,刘海立.重庆市PM2.5浓度时空变化特征分析研究[J]. 环境与发展, 2018,30(1):159-160. 王嫣然.北京市PM2.5浓度时空变化特征及影响因素分析[D]. 北京:北京林业大学, 2016. 李松,罗绪强,徐红勤.基于GIS的贵州遵义市PM2.5污染时空特征及变化分析[J]. 环境工程, 2015, 33(11):63-66. 任才溶,基于并行随机森林的城市PM2.5浓度预测[D]. 太原:太原理工大学, 2018. 苏筱倩,安俊琳,张玉欣.基于支持向量机回归和小波变换的O3预报方法[J].中国环境科学,2019,39(9):3719-3726. 顾康康, 祝玲玲.合肥市主城区PM2.5时空分布特征研究[J]. 生态环境学报, 2018, 27(6):1107-1112. 杨云,付彦丽.关于空气中PM2.5质量浓度预测研究[J]. 计算机仿真, 2016, 33(3):413-418. 杨洋,陈春,高梦,等.合肥市空气中PM2.5的计量分析[J]. 河北北方学院学报(自然科学版), 2016, 32(11):50-55. 施悯悯,张庆国,张浩,等.合肥市大气能见度变化特征及其影响因素[J].应用生态学报,2017,28(2):485-492. 潘本锋, 汪巍, 李莉娜. 我国典型城市PM2.5空间分布均匀性分析[J]. 环境工程, 2015, 33(5):109-113. 李会霞,史兴民. 西安市PM2.5时空分布特征及气象成因[J]. 生态环境学报, 2016, 25(2):266-271. 王云中. 基于神经网络的PM2.5浓度预测研究与实现[D].西安:西安电子科技大学,2018. 殷国栋. GASA-BP神经网络在安徽省工业用电量预测中的应用研究[D].合肥:安徽建筑大学,2018. 王敏,邹滨,郭宇,等.基于BP人工神经网络的城市PM2.5浓度空间预测[J].环境污染与防治,2013,35(9):63-66,70. 王惠琳,胡树根,王耘.基于模拟退火遗传算法优化的BP网络在质量预测中的应用[J].轻工机械,2011,29(4):26-31,35. 马健,盛魁,朱庆友,等.基于遗传模拟退火三次指数平滑模型的中药材价格预测研究[J].佳木斯大学学报(自然科学版),2017,35(5):857-860,874. 李慧敏.基于三次指数平滑模型的雾霾天气预测[J].中国环境管理干部学院学报,2017,27(3):52-55,74. 张海霞,程先富,陈冉慧.安徽省PM2.5时空分布特征及关键影响因素识别研究[J].环境科学学报,2018,38(3):1080-1089. 崔淑华,倪艳姝.城市主要气体污染物与PM2.5相关性建模分析[J].森林工程,2016,32(2):65-68. 王学梅,陈滔,张庆国.PM2.5浓度智能数据分析及预测系统:2019SR0976437. V1.0[P]. SGAMBI L, GARAVAGLIA E, BASSO N, et al. Monte Carlo simulation for seismic analysis of a long span suspension bridge[J]. Engineering Structures, 2014,78:100-111. 骆勇鹏,黄方林,刘景良,等.基于有限测试数据的结构响应不确定性量化分析[J].铁道科学与工程学报,2018,15(12):3217-3224. 刘庚,毕如田,张朝,等.某焦化场地苯并(a)芘污染空间分布范围预测的不确定性分析[J].环境科学学报,2013,33(2):587-593. 张剑波,江泽友,房俊,等.Kappa统计量和Bland-Altman分析法在HbA1c试剂盒一致性评价中的应用[J].数学的实践与认识,2019,49(20):167-175. -
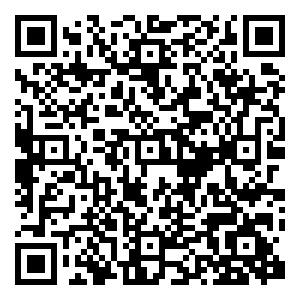
计量
- 文章访问数: 143
- HTML全文浏览量: 15
- PDF下载量: 15
- 被引次数: 0