CONSTRUCTION OF LightGBM WASTE PRODUCTION PREDICT MODEL IN A SCENARIO OF ZERO-WASTE CITY
-
摘要: 中国于2018年提出"无废城市"建设试点工作方案。通过对某试点研究区域调研发现,近年来当地生活垃圾产量呈现高出城市人口发展速度,且呈非线性快速增长趋势。传统的预测手段已经无法满足当地垃圾产量的精细化管理需求,难以将当地垃圾处理能力的发展与产量增加的趋势相协调。因此,基于社会多元数据,构建对研究区域整体垃圾总产量预测的模型研究方案。通过将灰色关联分析算法与LightGBM机器学习算法结合,获得了多元社会数据中与研究区域垃圾产生增长关联最为密切的几类特征数据,以进行机器学习模型构建与交叉验证调优,获得了MAE为1.48,MAPE为15.42%的生活垃圾产量精准预测模型。最终利用该模型预测,2025年该地的生活垃圾产量将达到17.23万t/a。Abstract: In 2018, China put forward a pilot project to construct Zero-Waste City. Through the investigation of the research area of one of the pilot cities, it was found that the domestic waste produced in this area showed a trend of rapid non-linear growth in recent years. Traditional predicting methods can no longer meet the expected delicacy management demand of local waste production. It is challenging to coordinate the development of local waste treatment capacity with the production increasing. Therefore, based on the urban multi-social data with the annual unit, a model research plan for predicting the overall waste production of the study area was proposed. Combining of the grey relational analysis algorithm and LightGBM machine learning algorithm can get several types of feature data in the multi-social data, which are most closely related to the growth of waste generation in the study area. The machine learning model construction and cross-validation tuning to obtain the domestic waste production prediction model with an MAE of 1.48 and an MAPE of 15.42%. Finally, the model predicted that domestic waste production in 2025 will reach 172,300 tons/year.
-
Key words:
- zero-waste city /
- domestic waste /
- production predict /
- grey relevance analysis /
- lightGBM
-
[1] SINGH J, LAURENTI R, SINHA R, et al. Progress and challenges to the global waste management system[J]. Waste Management & Research, 2014, 32(9):800-812. [2] 马梦蝶. "双碳"目标下上海市"无废城市"建设的协同路径研究[J]. 智能建筑与智慧城市, 2022(1):37-39. [3] LIU B C, ZHANG L, WANG Q S. Demand gap analysis of municipal solid waste landfill in Beijing:based on the municipal solid waste generation[J]. Waste Management, 2021, 134:42-51. [4] LIVANI E, NGUYEN R, de NZINGER J, et al. A hybrid machine learning method and its application in municipal waste prediction[J]. Lecture Notes in Computer Science, 2013, 7987:166-80. [5] AYELERU O O, NTULI F N O F. Municipal solid waste generation and characterization in the city of Johannesburg:a pathway for the implementation of zero waste[J]. Waste Management, 2018, 79:87-97. [6] KARIMI-JASHNI. S A A. Verifying the performance of artificial neural network and multiple linear regression in predicting the mean seasonal municipal solid waste generation rate:a case study of Fars province[J]. Waste Management, 2016, 48:14-23. [7] WANG P Y, ZHANG H, JIANG Y, et al. Prediction of Municipal Solid Waste Production in Dalian[J]. Environmental Sanitation Engineering, 2019. [8] LIN K S, ZHAO Y C, TIAN L, et al. Estimation of municipal solid waste amount based on one-dimension convolutional neural network and long short-term memory with attention mechanism model:a case study of Shanghai[J]. Science of The Total Environment, 2021, 791:148088. [9] 张旺. 基于Elman神经网络的城市生活垃圾清运量预测模型研究[D].武汉:湖北工业大学, 2017. [10] 赵天瑞. 无废城市典型场景下的生活垃圾动态模型构建及运输模式优化[D].哈尔滨:哈尔滨工业大学. [11] 曹飞飞. 灰色系统理论在粮食产量预测中的应用[J]. 数学的实践与认识, 2017,47(13):310-312. [12] ANDRES B, GORKA U, PEREZ J M, et al. Smart optimization of a friction-drilling process based on boosting ensembles[J]. Journal of Manufacturing Systems, 2018, 48:108-121. [13] 姜丽蓉, 李卓, 杨晓芳. 基于多种时间预测模型的组合对成都市垃圾生产量的预测[J]. 黑龙江科学, 2021, 12(6):32-33. [14] 李壮年, 储满生, 柳政根, 等. 基于机器学习和遗传算法的高炉参数预测与优化[J]. 东北大学学报(自然科学版), 2020,41(9):1262-1267. 期刊类型引用(3)
1. 杨延梅,舒辉秀,周奇,赵彤,包为磊. 基于灰色系统的城市生活垃圾产生量预测研究. 环境工程技术学报. 2024(03): 1048-1055 . 百度学术
2. 高帅,秦蔚,李同成. 大数据视野下的垃圾处置量关联分析——以成都市为例. 城市管理与科技. 2024(05): 68-72 . 百度学术
3. 胡翩,陈孝杨,牛经纬,华红梅. 基于省域尺度工业固废产量预测模型研究. 喀什大学学报. 2023(06): 47-51 . 百度学术
其他类型引用(7)
-
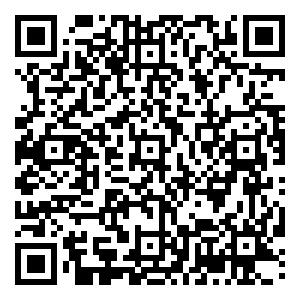
计量
- 文章访问数: 318
- HTML全文浏览量: 29
- PDF下载量: 9
- 被引次数: 10