CONSTUCTION AND COMPARATIVE ANALYSIS OF WATER QUALITY PREDICTION MODELS OF THE SANMENXIA RESERVOIR OF THE YELLOW RIVER
-
摘要: 水质预测是水污染防治的重要组成部分,为了提高黄河干流三门峡水库的水质预测精度、完善预警机制,选取pH、DO、NH3-N、TP以及CODMn 5项水库水质监测指标进行水质的精确预测。构建VMD-SSA-LSTM模型和VMD-SSA-SVR模型,采用变分模态分解(VMD)对河流水质数据进行去噪处理,选择麻雀搜索算法(SSA)分别对长短期记忆人工神经网络(LSTM)和支持向量回归机(SVR)的模型参数进行优化,并通过平均绝对值误差(MAE)、平均偏差误差(MBE)、均方误差(MSE)、均方根误差(RMSE)指标对2个模型的预测效果进行对比。结果表明:VMD-SSA-SVR模型的预测结果4项误差指标值均小于VMD-SSA-LSTM模型的预测结果,说明VMD-SSA-SVR模型对研究河流水质变化情况具有更加准确的预测效果。该研究丰富了黄河流域三门峡段河流水质预测模型方面的研究,为黄河流域生态保护和高质量发展提供了技术参考。
-
关键词:
- 水质预测 /
- 变分模态分解(VMD) /
- 麻雀搜索算法(SSA) /
- 长短期记忆人工神经网络(LSTM) /
- 支持向量回归机(SVR)
Abstract: Water quality prediction is an important part of water pollution prevention and control. To improve the accuracy of water quality prediction and the early warning mechanism of the Sanmenxia Reservoir on the mainstream of the Yellow River, five reservoir water quality monitoring indicators including pH, dissolved oxygen, ammonia nitrogen, total phosphorus and permanganate index, were selected for accurate prediction of water quality. The VMD-SSA-LSTM model and VMD-SSA-SVR model were constructed. The variational mode decomposition (VMD) was used to denoise the river water quality data. The sparrow search algorithm (SSA) was selected to optimize the model parameters of long short-term memory artificial neural network (LSTM) and support vector regression (SVR), and the prediction effects of the two models were compared by mean absolute error (MAE), mean bias error (MBE), mean square error (MSE) and root mean square error (RMSE). The results showed that the four error index values of the prediction results of the VMD-SSA-SVR model were smaller than that of the VMD-SSA-LSTM model, indicating that the VMD-SSA-SVR model had a more accurate prediction effect on river water quality changes. This study enriches the research on the river water quality prediction model in the Sanmenxia section of the Yellow River Basin, and provides technical reference for ecological protection and high-quality development of the Yellow River Basin. -
[1] 姜北, 邱林, 郑志宏. 水质预测方法研究综述[J]. 农业与技术, 2016,36(23):68-69. [2] 黄燏, 阙思思, 罗晗郁, 等. 长江流域重点断面水质时空变异特征及污染源解析[J]. 环境工程学报, 2023,17(8):2468-2483. [3] 陈鼎豪, 郑文丽, 王骥, 等. 简化一维水质模型在突发水污染事故模拟预测中的应用[J]. 环境工程学报, 2021,15(10):3199-3203. [4] 秦文虎, 陈溪莹. 基于长短时记忆网络的水质预测模型研究[J]. 安全与环境学报, 2020,20(1):328-334. [5] 陈肖, 张强, 姚年春. 基于VMD-IELM的淮安市二河溶氧量预测研究[J]. 信息技术与信息化, 2023(7):138-140. [6] 李建文, 孟连子, 刘娜, 等. 基于EEMD-SVR的渔业养殖水质预测模型[J]. 天津理工大学学报, 2018,34(5):14-19. [7] 王军, 高梓勋, 朱永明. 基于CNN-LSTM模型的黄河水质预测研究[J]. 人民黄河, 2021,43(5):96-99. [8] 赵隆, 温冠儒, 刘志成, 等. 基于参数优化的VMD-SVD和LSTM的输电杆塔倾斜状态识别[J]. 中国电力, 2023,56(12):217-226. [9] 何勇,张开雯. 基于EMD-SSA-LSTM模型的城市轨道交通站点客流预测[J]. 武汉理工大学学报(交通科学与工程版), 2024,48(5):829-835. [10] 刘辉, 李侯君, 刘雨薇, 等. 基于VMD和GWO-SVR的电力负荷预测方法[J]. 现代电子技术, 2020,43(23):167-172. [11] 马玉轩. 基于VMD的时频注意力TCN神经网络短期电力负荷预测[D]. 赣州:江西理工大学, 2022. [12] MIAO Q Y, SHU Q X, WU B, et al. A modified complex variational mode decomposition method for analyzing nonstationary signals with the low-frequency trend[J]. Sensors, 2022,22(5):1801. [13] 卿宗胜, 高云鹏, 吴聪, 等. 基于自适应VMD和改进功率谱估计的球磨机负荷特征提取[J]. 仪器仪表学报, 2020,41(5):234-241. [14] 向玲, 李京蓄, 王朋鹤, 等. 基于VMD-FIG和参数优化GRU的风速多步区间预测[J]. 太阳能学报, 2021,42(10):237-242. [15] XUE J K, SHEN B, PAN A Q. A hierarchical sparrow search algorithm to solve numerical optimization and estimate parameters of the carbon fiber drawing process[J]. Artificial Intelligence Review, 2023,56(1):1113-1148. [16] 崔忠捷. 基于SSA-LightGBM耦合模型的城市降雨径流实时预测研究[D]. 重庆:重庆大学, 2021. [17] 韩婧. 基于SSA-ELM的超短期风功率预测及其并网调度[D]. 郑州:华北水利水电大学, 2020. [18] 胡威, 张新燕, 李振恩, 等. 基于优化的VMD-mRMR-LSTM模型的短期负荷预测[J]. 电力系统保护与控制, 2022,50(1):88-97. [19] MA X L, TAO Z M, WANG Y H, et al. Long short-term memory neural network for traffic speed prediction using remote microwave sensor data[J]. Transportation Research Part C-Emerging Technologies, 2015,54:187-197. [20] 宋先知, 姚学喆, 李根生, 等. 基于LSTM-BP神经网络的地层孔隙压力计算方法[J]. 石油科学通报, 2022,7(1):12-23. [21] 谭海旺, 杨启亮, 邢建春, 等. 基于XGBoost-LSTM组合模型的光伏发电功率预测[J]. 太阳能学报, 2022,43(8):75-81. [22] 王宇胜, 陈德旺, 蔡俊鹏, 等. 基于LSTM-SVR的锂电池健康状态预测研究[J]. 电源技术, 2020,44(12):1784-1787. [23] 张琳, 汪廷华, 周慧颖. 基于群智能算法的SVR参数优化研究进展[J]. 计算机工程与应用, 2021,57(16):50-64. [24] 刘杰, 李佟, 李军. 基于改进支持向量回归机的污水处理厂出水总氮预测模型[J]. 环境工程学报, 2018,12(1):119-126. 期刊类型引用(0)
其他类型引用(1)
-
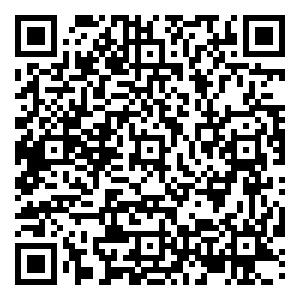
计量
- 文章访问数: 206
- HTML全文浏览量: 34
- PDF下载量: 14
- 被引次数: 1